2 Banking on Experiments?
Martin Dufwenberg1 April 2013
This paper started as a lecture prepared for an internal seminar on banking issues organized by the Norwegian Ministry of Finance in Oslo on september 24, 2012. I thank Price Fishback, Michael Kirchler, Hubert Kiss, Johanna Möllerström, Asaf Plan, Stefanie Ramirez, David Rietzke, Alec Smith, Morten Søberg, Stefan Trautmann, Tiemen Woutersen, and participants at seminars at the Universities of Arizona and Gothenburg for helpful comments.
Abstract: How can laboratory experiments, as well as insights from behavioral finance, help us understand financial crises as well as the usefulness of various policy responses? I attempt to provide answers, with a particular emphasis on banking crises. I also provide concise overviews of experimental economics and behavioral finance, covering methods and insights including a critical evaluation of external validity.
I. Introduction
How can laboratory experiments, as well as insights from behavioral finance, help us understand financial crises as well as the usefulness of various policy responses? I attempt to provide answers, with a particular emphasis on banking crises. I also provide concise overviews of experimental economics and behavioral finance, covering methods and insights including a critical evaluation of external validity.
Section II sets the stage: I first introduce the substantive issues – banks, crises, policy – that motivate the report, and then introduce the disciplines of experimental economics and behavioral finance, covering methodology, results, and relevance for banking. Section III concerns how experiments can inform understanding of banking crises: I identify themes that experiments can (or cannot) address, and then follow two main tracks that emerge as relevant. Part IV sums up and draws policy lessons.
II. Background
II-A. Banks, Crises, Policy
The field of finance is concerned with value and economic exchange in regards to streams of income over time.2 How will financial assets be priced? Who lends to whom on what terms?
The term financial crisis is used differently by various scholars; see Mishkin (1992) for an interesting discussion. His preferred definition requires that an unexpected change in some economic variable skews real investment incentives away from what would be most productive (because of so-called adverse selection or moral hazard). Many take a less structured/more intuitive approach, equating a crisis with large unexpected losses of value. Examples include exchange rate collapses, stock market crashes, bursting asset price bubbles, sovereign government defaults, and bank failures. The word “crisis” is suggestive that such episodes often involve major disruptions in people’s lives, as savings evaporate or layoffs occur (say by a distressed bank or government) or, if falling demand and failing businesses reinforce each other, a recession or depression ensues. Avoiding financial crises may be an important policy objective if these episodes are considered grim.
This report is especially concerned with banking crises, where banks enter financial distress. Following Calomiris (2008), this may happen for two different reasons:
Banking crisis reason #1: Bank runs
Banking crisis reason #2: Insolvency
Bank runs occurs when suddenly so many depositors decide to withdraw their savings that a bank finds itself illiquid. Bank runs are sometimes called banking panics (especially if all banks face runs). Insolvency occurs when the value of a bank’s assets fall enough that it cannot meet its obligations, for example if a real estate market collapses and people default on mortgage loans.
Scholars differ in their outlook on whether bank runs or fundamental shocks that cause insolvency are more important (and also whether one may cause the other). Calomiris (2008) explains how the idea that bank runs are key gained popularity through Friedman & Schwartz’ (1963) classic book A Monetary History of the United States. However, his own view, based on a careful scrutiny of bank balance sheets, is that shocks causing insolvency are more important. Calomiris does not argue that runs do not happen, only that they are not associated with great social costs.3 But many other economists seem to attribute a much larger role to runs as being socially costly and important for understanding banking crises; see, for example, the remarks of Larry Summers (2008) in his Arthur M. Okun Lecture “Learning from and Responding to Financial Crisis” delivered at Yale (check after 43 minutes). Lack of consensus is reflected also in the (FCIC/Angelides commission’s) Financial Crisis Inquiry Report (2011), which reached partly split conclusions (along partisan lines).
The explanations of banking crises are important because the optimal policy response (e.g. whether or not to use deposit insurance) differs depending on the underlying reason. The controversy offers a nice starting point when considering whether and how a novel approach may inform the debate!
II-B. Experimental Economics
Experimental economics (EE) differs from other subfields of economics like finance, public economics, labor, or social choice in that it is characterized by its method rather than its topic. This report belongs to finance by its focus on banking crises and to EE as it concerns using experiments. In this section I introduce the methods and contributions of EE. I start out describing a particular experiment which helps illustrate subsequent points.
The Bubbles Example
Many historical episodes involve dramatic price hikes of some commodity or asset, followed by a sudden collapse. Perhaps most famous is the Dutch “tulipmania,” where prices of tulip bulbs reached levels several times the yearly salary of an average wage earner, and then suddenly crashed in february 1637.4 More recently we have the development of the Nasdaq around 2000, and the US real estate market 2003-10. The patterns are often seen to involve “bubbles,” a term suggesting that prices exceed the expected present value of all future returns to a given asset (the “fundamental value”). Commentators, who view some asset price development as a bubble often use terms like hysteria, mania, panics, or (Alan Greenspan’s) “irrational exuberance,” as in the titles of Kindleberger’s (2001) and Shiller’s (2000) books.
It is, however, treacherous to evaluate the degree of madness of a market, as it is difficult to know what the fundamental value really should be. In fact, some economists call to question the bubble/crash terminology altogether, arguing that what at first glance seem hysterical may on closer scrutiny have sensible fundamental explanations (say, changes in discount rates or profit expectations). See, for example, Garber (2000).
The situation is imperfectly understood and hotly debated. Are bubbles common or rare? The issue is policy relevant. Bubbles and crashes would seem to generate huge shifts of wealth, and may affect when and what investments are made. If investment occurs in industries that are valued above fundamental value, then it may not always be the most lucrative investment projects that are embarked on. Whether bubbles occur moreover matters for evaluating policy, for example the effect of turnover taxes in financial markets (so called “Tobin taxes”) or the effect of monetary or fiscal policy.
Experiments offer a novel way to illuminate the issue. In the lab, one can construct a market with trading rules that share key features with a stock exchange, but where the dividend structure is controlled by the experimenter such that a fundamental value can be calculated. That value can then be compared to the prices generated in the experimental market, so that insights on the bubbles-vs-fundamentals issue can be drawn by analogy.
The pioneering contribution along these lines is Smith, Suchanek & Williams (1988) (SSW). A large related literature has followed. I’ll describe (part of) of a design used by Dufwenberg, Lindqvist & Moore (2005) (DLM), which is comparable.
DLM study experimental markets each involving six traders (students, recruited at Virginia Polytechnic Institute, where the experiment was run). Trade took place through continuous-time trading according to rules akin to those in stock markets, so-called “double-auction” rules, in which presumptive sellers place and revise asks, while buyers place and revise bids, and anyone can accept the lowest ask/highest bid at any time.
A market involved ten periods. The financial assets traded had a life corresponding to those ten periods. In each period trading was allowed for two minutes. After each period, whoever owned the financial asset being traded was entitled to a stochastic dividend, which was either 0 or 20 US cent each with probability 1/2. This was publicly made known to everyone.
An assets fundamental value can be calculated looking at the expected value of dividends to come (abstracting away from risk premia). With one period left, an asset should be worth 10 cents (= ½ x 0 cents + ½ x 20 cents); with two periods left, it should be worth 20 cent (= 2 x [½ x 0 cents + ½ x 20 cents]); with three periods left, it should be worth 30 cent; etc.
The trading prices can be compared to these fundamental values. The spirit of the results is illustrated in Figure 2.1, based on data from a selected typical market. Note that the horizontal axis re-starts the period count after 10. For now, focus on market 1, the leftmost of the four:
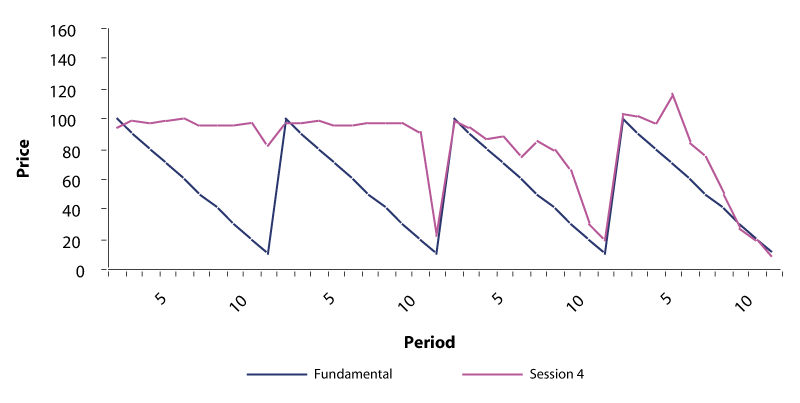
Figur 2.1 Observed mean prices, alongside fundamental value
The straight line that descends linearly from 100 (cents) to 10 indicates fundamental value. The other line indicates average price in the market. One can note a distinct bubble. When the market starts trades occur on average slightly below fundamental values, but they soon exceed them, eventually substantially. It seems natural to draw the conclusion that bubbles may be common in financial markets since they appeared in this design. (However, be warned that this conclusion will be somewhat qualified in section III-C below!)
Treatments
Experiments often involve treatments: multiple versions of a design, typically differing just in a single aspect, so that one can isolate the effect of that aspect. To exemplify, use The Bubbles Example: Suppose someone were interested in exploring the effect of trader fatigue on bubble formation. That researcher may complement the DLM design with an additional treatment identical in every respect except that the session in question were run late at night.
Control offered by treatments is a main selling point of experiments, but not all experiments have treatments. The Bubbles Example can illustrate. No change of design-feature was described (except the thought-up one of the previous paragraph), yet something useful was produced namely actual price data to be compared to theoretical fundamental values.
Why run experiments?
Seventeen years old, the Handbook of Experimental Economics (Kagel & Roth, eds., 1995) remains a good guide to its topic. In the introductory chapter Al Roth explains how experiments are run for different reasons (largely reflecting why empirical studies are done more generally):
Reason #1: Speaking to theorists
Reason #2: Searching for facts
Reason #3: Whispering in the ears of princes
A propos #1: Economic theorists tell stories about how thought-up worlds work. This may be valuable per se, but the scholarship gains relevance if it is empirically relevant. Experiments can evaluate that and The Bubbles Example illustrates: Let the theoretical story be that market prices reflect fundamental values. The experiment tests that proposition.
#2: Experiments sometimes just document interesting data (possibly to be addressed by future theory). The Bubbles Example illustrates, if its interpretation is tweaked. Suppose one did not refer to any theory, that one were merely curious about what patterns of trade emerge. The experiment produces data. Another, perhaps more interesting, example would be an experiment recording treatment effects (e.g., the trader-fatigue design mentioned earlier).
#3: The idea would be to offer policy advice. The Bubbles Example again illustrates; recall the comments on potential relevance for evaluating turnover taxes. That angle is somewhat indirect though. To get a more clearly relevant example, imagine a new treatment, including a laboratory turnover tax, to be compared to the treatment previously described.
Contributions
In the Handbook’s introductory chapter, Roth also gives a nice overview of the early history of EE (≈1940-1970). Contributions concerned three areas: (i) individual decisions, (ii) game theory, (iii) market experiments. This tri-part classification works for organizing research to this day.5 Area (iii) was already illustrated, through The Bubbles Example. Examples related to (ii) come in in section III-B, as bank run experiments belong to the category.
What is the most interesting recent development in EE? Different scholars would surely stress different matters. In my own opinion it concerns experimentation related to the growing field of behavioral economics, which incorporates insights from pychology into economic analysis. Experiments can document empirical relevance of psychological aspects that economists have traditionally abstracted away from, and they can be used to test behavioral theory. For entries to parts of the literature, see Camerer (2008). Section III-C below may be seen to contain an example, as a behavioral hypothesis is explored there.
Vernon Smith’s Nobel
Space constraints and desire to focus imply that I will not survey EE in much more detail. However, I would be remiss unless I commented on the scholarship of Vernon Smith, which landed him the Nobel Memorial Prize in 2002 (shared with Daniel Kahneman):
A basic tenet of much economic scholarship is that one may understand markets with many buyers and sellers such that price and quantity is given by the intersection of demand and supply curves. That outcome is often “efficient,” meaning that no potential gains from trade go unexploited. It is important to assess whether or not this story is empirically relevant.
Smith’s (1962) classic study of double-auction markets, in which buyers and sellers are active posting public bids and asks just like in a stock exchange, tackles that task. Trade took place in a commodity for which Smith “induced” valuations/production costs. Buyers were told that if at the end of a trading session they owned a unit, they would get paid a certain amount of money. Sellers were told that if they produced and sold a unit they would have to pay a certain cost. Controling valuations/costs this way, the classical market predictions become testable.
The data matched the theory well. Later research has shown that requirements to get efficient outcomes are even less restrictive than theory would suggest, as the markets work well even if the number of participants is small, say two or three buyers or sellers. Smith and others have also performed research on how a wide variety of other market forms (e.g. monopoly markets, posted offer markets, duopolistic competion, auctions) work. More often than not, the outcomes are rather efficient, although in that regard the double auction is hardly surpassed.6
Recall The Bubbles Example. The design involved double-auction trading rules. In light of what is known about the efficiency properties of such trading rules under conditions with induced values, it is remarkable that bubbles occur when experimental financial assets are traded.
II.C. Behavioral Finance
“[B]ehavioral finance uses insights from psychology to understand how human behavior influences the decisions of individuals and professional investors, markets and managers.” The quote is from a recent textbook by Ackert & Dawes (2010, p. xxvi). I’ll now describe the field, drawing in large part on the survey by Barberis & Thaler (2003) (B&T).7
Classical finance is built on the Efficient Market Hypothesis (EMH), according to which market prices track fundamental values (= the present value of expected returns). Texts motivating behavioral finance (BF) often describe data at odds with the EMH. For example, B&T (section 2.3.1) describe how two companies “agreed to merge their interests on a 60:40 basis” (and so face proportional returns in the future) and yet did not exhibit a 60:40 price-of-equity ratio. Another case where the EMH is violated is The Bubbles Example (II-B).
B&T argue that there are two “building blocks” to BF:
Building block #1: Limits to arbitrage
Building block #2: Psychology
A common defense of the EMH is that if markets were not efficient rational investors would make profit through risk-less arbitrage, in the process making markets efficient. Building block #1 points out a variety of reasons why engaging in arbitrage may be difficult, or impossible. For example, arbitrage may require short-selling, which may be costly or not allowed. Or if there are “noise traders” in the market, people whose decisions cannot be described as rational (cf. De Long, Schleifer, Summers & Waldmann 1990), then it can be a rational response to not let fundamental value govern trade. The Bubbles Example can illustrate: If a rational investor anticipates a bubbles rising then, despite that fundamental value is lower than current prices, it would be rational to buy and not to sell. The idea would be to “ride-the bubble”, and get out just before it bursts. This may feed the bubble further.
Building block #2 becomes relevant once one rejects the EMH. What comes instead? A literature attempts to describe how notions from psychology shape investor strategy and pricing. B&T cover overconfidence, wishful thinking, base rate neglect, gambler’s fallacy, belief anchoring, availability heuristics, reference dependent utility, loss aversion, ambiguity aversion, sample size neglect, and hot hand phenomenon.8 I will here describe only the last two notions, as they have direct bearing on things to come (III-B). Take it from B&T (p. 1065):
Sample size neglect means that in cases where people do not initially know the data-generating process, they will tend to infer it too quickly on the basis of too few data points. For instance, they will come to believe that a financial analyst with four good stock picks is talented because four successes are not representative of a bad or mediocre analyst. It also generates a “hot hand” phenomenon, whereby sports fans become convinced that a basketball player who has made three shots in a row is on a hot streak and will score again, even though there is no evidence of a hot hand in the data [Gilovich, Vallone & Tversky (1985)]. This belief that even small samples will reflect the properties of the parent population is sometimes known as the “law of small numbers” [Rabin (2002)].
These phenomena have bearing on how to understand bubbles experiments. If traders see prices rise, they may simply believe this pattern will continue, so that it seems wise to buy, not to sell.9
III. Experiments on Banking
III-A. Roadmap
There is some controversy regarding how to explain, and avoid, banking crises. Experimental economists bring new tools to the discussion. This subsection attempts to identify areas where to dig; the following two subsection then explore what’s been done.
To get an anchor for the discussion, consider how – in a recent briefing titled “Banking Crisis Yesterday & Today” – Calomiris (2009a; cf 2009b) sums up his views:
This brief survey of the history of banking crises traces unusual bank fragility to risk-inviting microeconomic rules of the banking game established by governments, the most important of which have been rules that subsidize risk. Other destabilizing rules include limits on bank entry and the failure to establish a proper lender of last resort. The subprime crisis exemplifies the historical pattern all too well. Government subsidization of risky mortgages in the U.S. accelerated markedly in the years prior to the crisis. That along with prudential regulatory failures to prevent excessive risk taking allowed the mortgage risk binge of 2003-2007 to produce a worldwide financial collapse. As the U.S. gears up to respond to the subprime crisis with regulatory reforms, history suggests important lessons.
The passage reflects two ideas: First (between the lines), insolvency is a more important source of banking crises than bank runs (cf. II-A). Second, the reason is bad government policy causing behavior changes (a form of moral hazard).
I think it is fair to say that many economists would somewhat dispute the account. For example, in his 2008 Okun Lecture, Larry Summers, who helped engineer much policy, while not thinking about or engaging in discussion geared directly to Calomiris, stressed the importance of bank runs for understanding banking crises and discussed the need for government intervention in this connection rather than focused on the problems that government activity may have caused. My impression is that Summers would want to add riders to Calomiris’ account.
Who is right? I will not say. I consider whether experiments can illuminate the debate. It doesn’t take much reflection to see a reason why one may hope this could be done. History has no counterfactual! To test the empirical validity of Calomiris claim it would be useful to compare the existing record with what would have happened had government pursued other policies. It is impossible to change history, but one wonders: can lab experiments help?
Cul-de-sac
My answer, as regards getting direct evidence, is that this may prove difficult or impossible. Reflect on what it would take. The key problem, described in the previous paragraph, is lack of data regarding counterfactual circumstances. Lab experiments would seem to have a shot at providing that, as a virtue of the lab is that one can compare treatments. However, the real world may prove too complex to allow direct insights-by-analogy that way. History involved a very complex game, with bank managers, their employees with varying incentives, their customers with their lives and trade-offs and deposit decisions, and government with all its people involved. Anyone who reads the Financial Crisis Inquiry Report (2011) will see this clearly. It is hard to imagine a design that would shed light on the empirical relevance of claims such as Calomiris’ through an insight-by-analogy approach. That’s the bad news.
Ways to go
Now the good news: Experiments may inform the debate regarding how to understand and react to banking crises more indirectly, by evaluating the empirical relevance of various ideas that economists bring up when they engage in debate. That is, rather than attempt to evaluate historical events by creating grand-scale parallels, experiments can zoom in on isolated building blocks that economists argue matter and evaluate the relevance of those.
Section III-B considers the idea that bank runs cause crises. Economists have traditionally given substance to that via models where bank runs make sense. In particular, in a classic contribution Diamond & Dybvig (1984) (D&D) model a bank run as the occurrence of a “bad equilibrium” in a game admitting two equilibria. The other equilibrium is “good,” and involves no run. What if players always coordinate on that one? That would undermine the relevance of the D&D model. Lab experiments can help illuminate the issue. In the lab one may create a D&D world, and explore the conditions under which its bank run equilibrium is viable. Whatever findings obtain, it may affect the confidence with which scholars can argue that the D&D story for explaining bank runs is relevant.
Section III-C considers the insolvency explanation of banking crises. Calomiris claims that banking crises largely reflect insolvency due to government policies that stimulated excessive risk taking. Is that plausible? I will discuss ways to evaluate that.
III-B. Bank Runs
With 5700+ Google Scholar citations, Diamond & Dybvig’s (1983) (D&D) model of bank runs is a classic. Calomiris & Gorton (1991, p. 120) summarize the outlook that motivated the work:
[In the early 80s], theoretical work on banks and banking panics was aimed at addressing the following questions: How can bank debt contracts be optimal if such contracts lead to banking panics? Why would privately issued circulating bank debt be used to finance nonmarketable assets if this combination leads to socially costly panics? Posed in this way, explaining panics was extremely difficult.
D&D depict banking as game with one good equilibrium where people keep their money in the bank and let them grow with a profit, and a bad equilibrium where everyone panics and withdraws deposits so that banks run out of cash and collapse. Readers satisfied with that account may fast forward a few paragraphs; for others I illustrate D&D’s model through an example that highlights key features & intuitions.10
Consider a society with n individuals each of whom has 1 unit of money. There are three periods: 0, 1, 2. Technology permits investments made in period 0 to grow to R > 1 units in period 2. However, if an invested unit is pulled back in period 1, the value stays at 1 (it takes two periods for projects to bear fruit). All individuals make the investment in period 0 but a proportion t < 1 of “need money tomorrow” and are forced to pull their investments back at period 1; t is known by all beforehand although in period 0 no one knows who will be forced to pull back early. Let u(w) be the utility of getting w. Each individual gets expected utility
t × u(1) + (1-t) × u(R)
Individuals are risk averse: t×u(1)+(1-t)×u(R) < u(t×1+(1-t)×R), for all R > 1. There is scope for a bank to improve the outcome by acting as an intermediary between the individuals and the technology. Instead of investing directly in the technology, individuals become bank customers. Each deposit his unit in period 0, then chooses when to withdraw funds. By judicious choice of offered returns, the bank may provide valuable insurance.
I illustrate under the assumptions that n = 6, t = ½, R = 4, and u(w) = 2-2/w for all w ≥ 0.11 Using
½ × (2-2/1) + ½ × (2-2/4) = 3/4
Suppose a bank offers a return of 1+r if a withdrawal is made in period 1 and a return of (1-r)×4 if a withdrawal is made in period 2, so the bank breaks even when three-out-of-six customers withdraw early.12 A customer’s expected utility in period 0 is
½ × (2-2/(1+r)) + ½ × (2-2/((1-r)×4)
If, for example, r = 1/3 the expression becomes
½ × (2-2/(1+1/3)) + ½ × (2-2/((1-1/3)×4) =
= (1-3/4) + (1-3/8) = 7/8
Since 7/8 > 3/4, consumers are better off with the bank.13
The analysis so far assumed that only the three-out-of-six customers who need the money in period 1 withdraw early. The described pattern is a Nash equilibrium (meaning that each customer optimizes, given the behavior of others). The three-out-of-six customers who need money in period 1 obviously do what’s in their best interest. So do the other three, who each get (1-r)×4 = (1-1/3)×4 = 8/3, which is more than those who withdraw in period 1 get.
However – and here comes a key insight – the game has a second, bad, equilibrium in which all customers withdraw their money in period 1, whether they need the money or not. To see this, consider a customer who does not need money in period 1, but who believes the other five are all withdrawing then. Since 6×1 units were deposited in period 0, five withdrawals of the others in period 1, if served, would amount to 5×(1+r) = 5×(1+1/3) = 20/3. Since 20/3 > 6, there won’t be enough money in the bank to serve these five early withdrawers. Consequently, there will also be no money left for our customer if he waits till period 2. His best response is to withdraw in period 1 too.
This “bank run equilibrium” is inefficient since the investments never yield the desired return; no units get quadrupled. In fact, it is worse than just keeping the money in the mattress (not even investing in the first place), as in the bank run four-out-of-six customers get 1+r = 1+1/3 = 4/3, one customer gets 2/3, and one customer gets nothing;14 in expectation they each get 1, but they are risk averse and would have preferred to get 1 for sure.
Note the key role played by r > 0. On the one hand, it is through the ability to offer a period 1 positive return to early withdrawers that the bank acquires its raison-d’être, the ability to offer insurance to risk-averse individuals. On the other hand, once a sufficiently high r > 0 is in place, the possibility of a bank run comes alive.
R as in insolvency?
D&D’s bank run equilibrium can potentially explain how inefficient outcomes arise merely because economic agents come to coordinate on an inferior equilibrium, rather than because of some other adverse economic shocks. As indicated before, the empirical relevance is contested (e.g. Calomiris & Mason 1997, 2003; cf. II-A). However, to this day it is nevertheless often mentioned as highly relevant. Summers (2008) says that “this Diamond-Dybvig bank run metaphor has been very powerful in influencing financial policy in a wide range of situations”,15 which echoes his earlier (2000) remarks (in a Richard T. Ely Lecture) that while the likelihood of bank runs “is driven and determined by the extent of fundamental weaknesses” it is also an issue of “bank-run psychology” (p. 7). Another example: Paul Krugman explained in his New York Times column that he is “a Diamond and Dybvig guy” (Jan. 11, 2010).
In the D&D model, R is the returns offered by investments. It is useful to point out how to think of the link between R and the bank runs versus insolvency debate. In D&D’s model, R is a constant. In the real world, R is surely not constant; returns depend in complicated ways on lending practices, entrepreneur behavior, credit ratings, and government policy. R not being a constant is what the insolvency aspects of banking crises are all about! For example, when Calomiris blames government for the financial crisis, one way to think of this is that policy affected incentives so that behavior changes caused R to dip, a form of moral hazard. The D&D model, with R constant, abstracts away from all that, which allows D&D to explain how a banking crisis could arise for reasons that have nothing to do with insolvency.
Experiments
Lab experiments can inform the debate by evaluating the argument parts on which debaters build when they discuss how crises work. The D&D story is a key part, and lab experiments may evaluate its empirical relevance under various conditions. Several rather recent studies have done this. They involve games that in structure resemble the D&D model, although details differ. For the most part I will not attempt to describe these details, but will rather concentrate on main insights. I finally say something about angles that have not been addressed but which it would seem interesting to explore.
Madiès (2006)
A first finding is that most of the time neither of D&D’s equilibria occurs. Some subjects withdraw in period 1, some don’t. This is true even as the design allows subjects to gain experience, playing D&D games thirty times. Similar observations apply to most of the other studies to be discussed.
Madiès explores treatments that give subjects “more time to think before making further decisions” (p. 1854). He interprets this as “suspension of deposit convertability,” which D&D show may be helpful for avoiding runs. I find the interpretation questionable as in D&D’s theory, but not in the design, suspension entails reaching period 2 (where investment gains accrue) with denied opportunity of prior withdrawals. Anyway, Madiès’ design feature reduces period 1 withdrawals, suggesting that in some situations a cooling off period or bank holiday may reduce panics. That said, as Alec Smith told me: “I had a strong reaction to [that] policy implication. I think that closing banks can possibly have the opposite effect, that is to increase panics.”
Another set of results concern “deposit insurance” (guarantees of a certain level of return in case of a run). Madiès explores two “partial insurance” (75% & 25%) schemes. He reports that neither works, concluding that “only a total deposit coverage is effective in preventing bank runs” (p. 1855). One should note that Madiès scheme differs from D&D’s in nature. D&D prove (their section V) that in their model deposit insurance eliminates runs. Their scheme is self-financed, with a form of ex post depositor taxation based on the number of withdrawals. Madiès design, by contrast, involves outright transfers in case of many withdrawals and so does seem to not furnish a clear test of D&D’s result. At the same time, his structure may resemble some deposit insurance schemes used in practice more than D&D’s scheme does.
Garratt & Keister (2009) (G&K)
G&K have a 2×2 treatment structure. The first treatment variable concerns forcing a random subset of players to withdraw early, which G&K interpret as a “proxy for macroeconomic conditions” (p. 301) The second treatment variable concerns how many opportunities subjects have to withdraw during period 1, one or three (where after each opportunity subjects learn how many others withdrew); G&K say this “adds a realistic feature of banking: depositors have a period of time during which they can choose to withdraw their funds, and they are able to observe some information about actions of other depositors, for example, by noticing if a line is forming outside the bank” (p. 301). Across treatments, both D&D equilibria are always viable.
Forced withdrawals lead to more withdrawals by those not forced. G&K write: “the ability of people to coordinate on the payoff-dominant equilibrium [without a bank run] is sensitive to the presence of aggregate uncertainty about fundamental withdrawal demand, even when this uncertainty alone poses little or no threat to the solvency of the bank” and that the random forced withdrawals “mimic the type of uncertainty that is likely to be present under unfavorable macroeconomic conditions or in times of financial distress” (p. 311). It is interesting to compare this finding to Summers (2000) hypothesis that “bank runs … are not driven by sunspots” but rather “the extent of fundamental weaknesses” (p. 7).16 G&K’s design explores related ideas, but falls short of being able to evaluate the following: Suppose macroeconomic conditions go sour, and yet a particular D&D style banking games has its payoffs unchanged (unlike G&K’s games, where payoffs change alongside). Could it be that the tendency towards the bank run equilibrium is nevertheless enhanced? Put differently, G&K’s forced withdrawals not only add an element of uncertainty but also change the riskiness of not withdrawing as the expected number of early withdrawers change; the design doesn’t disentangle these effects.
As regards the treatments with multiple withdrawal opportunities during period 1, more actual period 1 withdrawals appear. G&K interpret this to “suggest that in countries where people have a history of exposure to financial crises, withdrawal behavior might depend on … the information flow regarding the withdrawal activity of others” (p. 311).
Schotter & Yorulmazer (2009) (S&Y)
S&Y are more interested in the dynamics of runs than in whether or not runs occur. They explore a design that differs from the previous two in that they consider cases where the bank is, at some point, for sure insolvent. Their games have a four-period structure, and in many cases all equilibria involve runs before period 4. The design is rather complicated, with treatments manipulating what subjects learn about others’ withdrawals, and whether or not “insiders” know about degree-of-solvency. S&Y also explore treatments with partial deposit insurance.
One treatment concerning information about others’ withdrawals is akin to G&K’s triple-withdrawal-opportunity; the results are comparable in that multiple opportunities to learn about others’ withdrawals increases withdrawals.
Another result, counter to Madiès’, is that partial deposit insurance “can help diminish the severity of bank runs” (p. 217). The (transfer) form of the insurance is similar to Madiès’.
S&Y also report results related to notions of transparency and insiders. One intriguing example is that the presence of insiders “mitigates the severity” of runs (p. 217). The reason seems related to the design-feature (not seen in Madiès or G&K) that some banks are insolvent, so depositors may interpret failure of insiders to withdraw as evidence their bank is solvent. Conceivably this supports the idea that insider trading can be useful (although I would suspect there are many counter-arguments to that idea not addressed by S&Y’s design).
Kiss, Rodriguez-Lara & Rosa-Garcia (KRR 2011, 2012)
KRR (2011) have a design in which two subjects act as depositors, interacting with each other and with a third computer-simulated early withdrawer depositor. Their two treatment variables concerns whether withdrawals are simultaneous or sequential with depositors informed of preceding decisions, and the degree of deposit insurance applied to payoffs (none, partial, or full; again, a transfer rather than a financed scheme). The “sequential setup decreases significantly the likelihood of bank runs” and “the main contribution” is that if “decisions are not simultaneous but sequential … deposit insurance decreases the likelihood of bank runs, but the effects of full and partial insurance are not significantly different” (p. 4). In a related study, KRR (2012) study many further variations on the information theme: three depositors act in sequence but the information about preceding decisions is altered. They find, for example, that runs are much less frequent whenever depositor 2 is informed of depositor 1’s decision (whatever it may be).
On the one hand, there may be limits to how much general insight one may take away from these results since the imposed structure, with an exogenously given queue of depositors making their decisions, is so special and stylized. (For example, how much sense does it really make, in general rather than in a queue, that depositors may observe others committing not to withdraw?) On the other hand, KRR’s studies very usefully highlight and alert us to how the prevalence of runs may depends on details concerning observability amongst the depositors. Their finding in this regard indicate that the topic may deserve more scrutiny, including (as Janos Kiss pointed out to me) how to endogenize the timing of the order in which depositors move.
Arifovic, Jiang & Xu (2011) (AJX)
By varying parameters in the D&D model, one can influence how many period 1 withdrawals it takes to make a depositor who is not forced to withdraw in period 1 nevertheless want to do so. For example, consider my example above, with n = 6, t = 1/2, R = 4, r = 1/3. Simple calculations show that a customer who believes that exactly three others withdraw in period 1 (the three customers forced to do so, plus one more) would be indifferent between withdrawing or not. If instead r > 1/3 [r < 1/3] he would prefer [not] to withdraw. It seems very plausible that changes of that sort, say changes that make r lower, make a bank run less likely. AJX report, essentially, that this intuition is supported by their data.
Klos & Sträter (2012) (K&S)
Coordination games, of which bank run games are a special case, have multiple equilibria. The “global games” approach (Carlsson & van Damme 1993) shows that, in many cases, if one incorporates uncertainty about a payoff parameter (think of R in a D&D game), and assume players get private signals of this parameter, then there may be a unique equilbrium (where behavior may change with the strength of the signal). See Dasgupta (2004), Goldstein & Pauzner (2005), and Rochet & Vives (2004) for applications to bank runs. There is an experimental literature on global games (see e.g. Heinemann, Nagel & Ockenfels 2004 study of currency attacks) and K&S (2012) take first steps testing global games related ideas in experimental bank run games. They compare the predictive power of global games theory vs. so-called level-k theory, declaring the latter winner (see Crawford, Costa-Gomes & Iriberri 2013, for an introduction to level-k theory, and note their remarks in section 7 concerning bank runs).
Contagion
The studies discussed so far concern runs in given banks. When there are several banks around there is an issue of bank run contagion in which runs at one bank, when observed by depositors in other banks, induce runs there. Two recent, very similar in structure, experiments by Brown, Trautmann & Vlahu (2012) (BTV) and Chakravary, Fonseca & Kaplan (2013) (CFK) are aimed at understanding the mechanics of bank runs in such setting. Their designs involve two banks; the customers of one make their decision to withdraw or not before those of the other bank. Some of the parameters of the environment (analogous, say, to my R above) are subject to stochastic shocks (which are small enough to not change the D&D style multiple equilibria feature of the games, but which move basins of attraction somewhat like in AJX). Treatments concerns whether or not the realizations of the shocks that concern the first bank are correlated with the shocks that apply to the second bank. The question is, to cite BTV (p. 3) “whether bank runs are only contagious when there are economic linkages between the banks.” Both studies report evidence that linkages/correlation fosters bank run contagion. Without linkages/correlation, the two studies reach somewhat different conclusions. BTT do not observe contagion, while CFK do (though to a more limited extent than with linkages/correlation).
Things to do
I offered critical remarks about various aspects of the studies I surveyed. Those comments implicitly suggest issues where more clarifying or corroborating evidence may be called for, and I will not say more about that here but rather bring up new themes.
D&D inspired a huge literature of follow-up theory. For example, while D&D restrict attention to simple demand-deposit contracts, Green & Lin (2003) show that other contracts may rule out equilibrium runs. It is beyond the scope of my report to summarize the literature but I would like to note that, surely, there must be exciting ideas developed that cry out for experimental testing.
D&D’s analysis starts with deposits already in the bank. There must have been an earlier period where the deposit decisions were made. Suppose that stage is explicitly added to the model. As van Damme (1994, p. 22) notes, it would seem that no bank run equilibrium could be sustained, as presumably the best response would be to not deposit in the first place.17 And if a deposit is made this might signal the intent to play the good equilibrium without a bank run, which may make others tag along. It may be fruitful to tests the empirical relevance of these ideas in an experiment, and I’m thinking about doing such research myself.
Finally, I wish to note two limitations that apply to all the papers I surveyed. First, D&D’s model embodies two key components: (i) It explains why banks are needed and formed (to provide a form of insurance); (ii) it explains bank runs in terms of coordination on an inefficient equilibrium. Much of the beauty of D&D’s work lies in how (i) and (ii) are inter-linked (via r > 0; cf. my earlier remarks). No such link is examined in experimental studies though; in fact, (i) is not addressed at all.18 Second, what are the key psychological factors involved in bank runs? Some would say “fear” or “panic”. Recall Summers’ (2000) remark on “bank run psychology.” If this is modeled by D&D, it is done somewhat soporifically, as an inefficient equilibrium. The experimental designs follow suit; the psychology of fear or panic is not really addressed.
III-C. Insolvency
What about the relevance of the insolvency story? In this section I will approach this issue from two different angles that concern, respectively, misprediction and moral hazard.
Sample size neglect, the hot hand phenomenon, and bubbles
As regards the 2007+ crisis, Calomiris points out that “[R]easonable, forward-looking estimates of risk were ignored” (quote from 2009c, p. 3; compare the longer analysis in 2009b, pp. 13-21).19 Part of the argument is that despite the writing on the wall, various market participants benefited from excessive loan origination. However, many participants were hurt as the market collapsed! It is puzzling that this could happen with such force, if there was writing on the wall. One key to explaining this would be if people were affected by sample size neglect & the hot hand phenomenon (recall II-C).
In section II-B, The Bubbles Example was presented chiefly to illustrate general themes discussed there. However, bubbles experiments may be relevant also for evaluating the empirical relevance of sample size neglect & the hot hand phenomenon which may make traders oblivious that a crash is imminent. With those goggles on, the presentation now picks up where The Bubbles Example ended. Prepare for a roller-coaster ride!
Recall the observation that bubbles appeared in DLM’s design, echoing earlier findings of SSW and others.20 It may seem natural to draw the conclusion, by analogy, that bubbles may be common in naturally occurring markets as well. However, a closer looks gives reason for pause. As seen at the time they published their paper, bubble & crash pricing patterns appear under a variety of circumstances, with one exception. If subjects have gained experience through participation in at least two preceding markets, then bubbles are significantly abated. Does this mean that the support from experiments for the view that bubbles & crashes occur is weak? That’s not clear. One could argue that the studies discussed so far did not settle the issue. In nearly all experiments either all or none of the market participants were experienced. By contrast, in naturally occurring markets, there is often a mixture of experienced and inexperienced traders. It is hard to know which result applies. Therefore, it would seem desirable to run experiments where the subjects in a market differ as regards their level of experience.
DLM report results from a lab experiment intended to shed light on the issue. Recall (from II-B) that a market design consisted of ten periods. Subjects actually interacted repeatedly in three such “market rounds”, and gained experience. (Note the distinction between a round and a period. A round consists of ten periods.) Then a fourth round was played, in which some (two or four, by now experienced) traders were replaced by inexperienced traders (that up till that point have solved crosswords, rather than interacted in any market). This fourth round is crucial. By comparing its data with data from rounds one and three, one can evaluate whether a mixed experience setting more resembles one with inexperienced or experienced traders.
Looking back at Figure 1, this time focusing also on the data from rounds three and four data, one sees that bubbles are common in the first round but abate by the third round. The bubbles do not return in the fourth round, where they resemble the prices of the third round (where all traders are arguably experienced) and do not resemble the prices of the first round (where all traders are inexperienced). DLM actually ran ten sessions and Figure 2.2 shows the data averaged across all of these, which underscores the pattern reported so far (this claim is supported also through formal statistical test, reported by DLM):
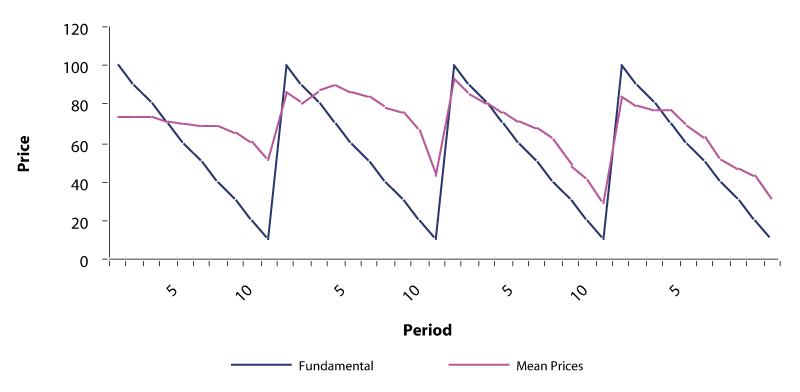
Figur 2.2 Observed mean prices, and fundamental value, across all sessions
DLM thus support the proposition that prices are fundamental, rather than bubbly, when market participants are heterogeneous as regards experience level. DLM sum it up: the “results may shift the burden of proof somewhat between those who believe in the madness of the market and the market fundamentalists. [The] results speak in favor of the latter position” (pp. 1735-6).
DLM turns on its head the conclusion of TheBubbles Example (II-A). However the story does not end here. Hussam, Porter & Smith (2008) (HPS) respond to DLM in a way that to an extent brings back the message of TheBubbles Example. HPS report data from a design which allows subjects to gain experience through repeat interaction. They “impose a large increase in liquidity and dividend uncertainty to shock the environment of experienced subjects who have converged to equilibrium, and this environment rekindles a bubble” (p. 924); “when important elements in the underlying market environment change for experienced subjects, a bubble can reignite” (p. 937). The burden of proof, alluded to by DLM as cited above, shifts back….
I’ll pretty much leave the bubbles topic here. I’d like to note, however, that research in the area is ongoing and new studies keep somewhat shifting the burden of proof in one or the other direction.21 I will indicate the content of only one study, which I find intriguing. Most experiments involve a linearly declining fundamental value. Kirchler, Huber & Stöckl (2012) (KHS) note that this feature differs from most naturally occurring contexts, where fundamental values are constant or increasing over time. Could this lead to subject confusion? To evaluate this possibility, they run a clever design in which the instructions refers to “stocks of a depletable gold mine” and this “significantly reduces mispricing and overvaluation” (p. 865).
KHS’ result could be read two ways. On the one hand, perhaps previous experiments exaggerated support for bubbles by using designs that subjects misunderstand. On the other hand, the result suggests that if fundamental values are (close to) common knowledge between subjects, then they do not bubble. This result may not tell us much if in naturally occurring markets fundamentals are unlikely to be common knowledge.
Moral hazard
Recall the remarks in section III-B, about “R as in insolvency”. In the D&D model R is constant, effectively ruling out moral hazard as a source of insolvency. However, one may consider banking games in which (some counterpart of) R is not constant. Theoretical examination may generate valuable insights, with subsequent experimental testing. This would be a new field of research,22 and I propose that thinking along these lines may be fruitful.
As I prepared my report, I discussed this topic with Stefanie Ramirez and David Rietzke, two PhD students at the University of Arizona. We came up with a project that fits the bill, and which we are excited about. This is early-work-in-progress but I’ll nevertheless sketch it, hoping to provide a flavor of how research in this area might look, and food for thought:
Historical records have been taken to suggest that deposit insurance often causes insolvency by inviting excessive risk-taking (see Calomiris 1990, 2008). How can this be understood? Suppose one writes down a model. A natural relevant game will have three players: bank customers, bankers, and some institution providing deposit insurance (say the FDIC). If customers deposit, then bankers can invest/give loans. Without deposit insurance, bankers are residual claimants once deposits are repaid; they go bankrupt if investments go sour or loans are not repaid. With deposit insurance, bankers are still residual claimants, but now customers’ losses are covered by deposit insurance. Compare the last two sentences, and reflect on how the monetary payoff to the bankers may well be the same in the two cases. If bankers maximize profit the outcome would seem to be the same for the bank, with or without deposit insurance, although the customers’ payoffs would vary greatly. (This abstracts away from how bankers may be more or less carefully monitored by customers or the FDIC, with or without insurance, a possibly important consideration.)
The model sketched so far does thus not furnish a compelling reason why deposit insurance creates enhanced incentives for excessive risk-taking. But this conclusion changes if one takes a relationship aspect between bankers and customers into account by applying reciprocity theory (Dufwenberg & Kirchsteiger 2004; cf. Rabin 1993). Customers who deposit will be viewed by the bank as kind, as deposits are what allow investment/lending. Without insurance, bankers will want to be kind in return, so they hold back on risk-taking.23 With deposit insurance, by contrast, excessive risk-taking is no longer unkind to the customers. (It may be unkind to whoever foots the insurance payments, but, according to reciprocity theory, bankers do not care if that party weren’t kind to them.)
Our plan is to develop theory + run lab experiments to test for empirical relevance.
IV. Conclusions
Reasonable men disagree on how to understand financial crises in general and banking crises in particular, and what to do to avoid or mitigate these events. How would history have changed had government policy, lending standards, credit rating practices, et cetera been different? One cannot go back in time and check. However, one can run lab experiments under controlled conditions. Can this be useful?
I presented bad news & good news. History is probably too complicated to be meaningfully revamped or modified in the lab, for purposes of insight-by-analogy. But as people argue about how to understand and shape financial history, they bring ideas to the table the empirical relevance of which might be meaningfully explored.
The debate on how to understand banking crises may be seen as centered on a bank runs versus insolvency issue. The bank run experiments, surveyed in section III-A, explore the empirical relevance of the story told by Diamond & Dybvig’s (D&D) model, which abstracts away from insolvency issues. The results are a mixed bag. It neither says D&D’s depiction of bank runs seem irrelevant nor super-compelling. There is moreover room for related work that may produce more robust insights.
What about the relevance of the insolvency story? In section III-B I approached this issue from two angles. First, I discussed the notions (developed by psychologists and emphasized in behavioral finance) of sample size neglect & the hot hand phenomenon, which may help explain why individuals might believe prices-will-keep-rising while neglecting important information about excessive risk-taking in the lending industry. Experiments on bubbles in financial markets furnish relevant test beds, and on balance somewhat support this idea. Second, I discussed (and proposed as in need of more research effort) moral hazard in banking, the use of models to examine that, and the possibility of running related experiments.
In the opening paragraph of my report I promised “a critical evaluation of external validity,” that is, the extent to which insights that hold in a lab environment have bearing on how to understand the external banking world in which one is ultimately interested. I already made my caveat that lab experiments hardly allow recreating history for insight-by-analogy purposes. Beyond that, in many cases I wouldn’t worry too much. For example, lab experiments with students should form adequate test beds for exploring the empirical relevance of sample size neglect & the hot hand effect among bankers, borrowers, and government officials. People are people! Lab experiments are also useful for testing theory, since by design one can often well represent the situation a theory depicts. Thus, for example, testing the empirical relevance of D&D’s logic in the lab makes sense.
How can lab experiments inform policy? In order to do well it is useful to first diagnose how the world works. Therefore, exploring for example the bank runs-versus-insolvency reasons for banking crises must have some policy relevance at least indirectly. As regards specific tools, consider deposit insurance. According to D&D, such schemes may be quite helpful for avoiding bank run equilibria. Some of the experiments (Madiès, S&Y, KRR) tested their effect in D&D designs, but the schemes used (conditional transfers rather than funded schemes) do not map well to what D&D have in their theory which makes it somewhat unclear what is tested. Besides, the results were pointing in different direction, for example concerning the usefulness of various degrees of partial insurance.
When reading these results, one must not forget that deposit insurance schemes may have terrible properties for reasons that could never be shed light on through any pattern of data. Take it from Calomiris (2008, p. 350; compare e.g. Calomiris 1990):
Empirical studies … conclude that deposit insurance and other policies that protect banks from market discipline, intended as a cure for instability, have become the single greatest source of banking instability. … Deposit insurance removes depositors’ incentives to monitor and discipline banks, and frees bankers to take imprudent risk.
D&D style bank run games, abstracting away from insolvency and moral hazard, do not address that but in section III-B I discussed the possibility of coming up with new games and experimental designs that do. Not much work has been done but I see the area as one where future research effort may be fruitful.
Next consider capital requirements (cash reserve ratios) that governments impose on banks. Are they useful? What level is best? Can lab experiments help evaluate that? I have not found any experiment done on the topic. Calomiris (2009c, p. 10) suggest that a regulatory rule of the following sort may be useful:
[V]ary capital and liquidity requirements over time in response to changes in macroeconomic and financial system circumstances. For example, during booms, minimum capital would be set higher, especially if a boom were occurring in which asset prices and credit were rising rapidly. Raising capital requirements on banks would discourage a protracted bubble from forming and create a larger equity cushion for banks if a bubble should burst.
His comments about “bubbles” suggest ways that asset market experiments of the sort I have covered could be useful in this connection. Finally, mutatis mutandis, introducing capital requirements to a D&D game influences its payoffs.24 It is natural to wonder what the empirical relevance may be and whether experiments could shed light on that.
The policy spectrum extends beyond deposit insurance and capital requirements. In preparing this report I found a lot of inspiration in the work of Charles Calomiris, so it it seems fitting to present his what-shouldn’t-have-been-done (in the US, 2003-07) and what-should-be-done lists (from Calomiris 2009a & 2009c), as regards avoiding banking crises:
What shouldn’t have been done:
Political pressures from Congress on the government-sponsored enterprises (GSEs) Fannie Mae and Freddie Mac, to promote ‘‘affordable housing’’ by investing in high-risk subprime mortgages
Lending subsidies for housing finance via the Federal Home Loan Bank System to its member institutions
Federal Housing Administration (FHA) subsidization of extremely high mortgage leverage and risk
Government and GSE mortgage foreclosure mitigation protocols that were developed in the late 1990s and early 2000s to reduce the costs to borrowers of failing to meet debt service requirements on mortgages, which further promoted risky mortgages
2006 legislation enacted to encourage ratings agencies to relax standards for subprime securitizations
What should be done: (1) regulatory taxes and reforms of resolution processes that would discourage too-big-to-fail protection of large, complex banks, (2) macro prudential regulatory authority to gauge overall risk in the financial system and structure dynamic capital and liquidity requirements accordingly, (3) elimination of leverage subsidies in housing, (4) rules to encourage OTC clearing in clearinghouses, (5) disclosure standards for OTC market participants, (6) improvements in the measurement of regulatory risk that would include market-based measures, (7) changes in the use of rating agencies’ opinions to discourage grade inflation, and (8) eliminating regulatory limits on the concentration of ownership in banks.
Might lab experiments be relevant for shedding light on the usefulness of the policies indicated? If I led my readers to consider that question natural, then I would rate my report a success.
REFERENCES
Ackert, L. & R. Dawes (2010), Behavioral Finance: Psychology, Decision-Making, and Markets, Southwestern.
Arifovic, J., J.H. Jiang & Y. Xu (2011), “Experimental Evidence of Bank Runs as Pure Coordination Failures,” mimeo.
Barberis, N. & R. Thaler (2003), “A Survey of Behavioral Finance,” in Handbook of the Economics of Finance, G.M. Constantinides, M. Harris & R. Stulz (eds.), Elsevier.
Bloomfield (2008), “Behavioral Finance”, The New Palgrave Dictionary of Economics, 2nd ed. S.N. Durlauf & L.E. Blume (Eds.), vol. 1, 438-444, Palgrave Macmillan.
Brown, M. & C. Zehnder (2007), “Credit Reporting, Relationship Banking, and Loan Repayment,” Journal of Money, Credit & Banking 39, 1883-1918.
Brown, M. & C. Zehnder (2010), “The Emergence of Information Sharing in Credit Markets,” Journal of Financial Intermediation 19, 255-78.
Brown, M. S. Trautmann & R. Vlahu (2012), “Contagious Bank Runs: Experimental Evidence,” DNB Working Paper 363, De Nederlandsche Bank.
Caginalp, G., D. Porter & V. Smith (1998), “Initial Cash/Asset Ratio and Asset Prices: An Experimental Study,” Proceedings for the National Academy of Sciences 95, 756-61.
Caginalp, G., D. Porter & V. Smith (2000), “Momentum and Overreaction in Experimental Asset markets,” International Journal of Industrial organization 18, 187-204.
Caginalp, G., D. Porter & V. Smith (2001) “Financial Bubbles, Excess Cash, Momentum, and Incomplete Information,” Journal of Psychology & Financial Markets 2, 80-99.
Calomiris, C. (1990) “Is Deposit Insurance Necessary? A Historical Perspective,” Journal of Economic History 50, 283-952.
Calomiris, C. (2008), “Banking Crises”, The New Palgrave Dictionary of Economics, 2nd ed. S.N. Durlauf & L.E. Blume (Eds.), vol. 1, 348-352, Palgrave Macmillan.
Calomiris, C. (2009a) “Banking Crisis Yesterday and Today,” briefing to the Pew Financial Reform Task Force.
Calomiris, C. (2009b) “The Subprime Turmoil: What’s Old, What’s New, and What’s Next,” Journal of Structured Finance 15, 6-52.
Calomiris, C. (2009c) “Prudential Bank Regulation: What’s Broke and How to Fix It,” unpublished manuscript.
Calomiris, C. & G. Gorton (1991), “The Origin of Banking Panics: Models, Facts, and Bank Regulations,”, in Financial Markets & Financial Crises, R.G. Hubbard (ed.), University of Chicago Press.
Calomiris, C. & C. Kahn (1991), “The Role of Demandable Debt in Structuring Optimal Banking Arrangements,” American Economic Review 81, 497-513.
Calomiris, C. & J. Mason (1997), “Contagion and Bank Failures During the Great Depression: the June 1932 Chicago Banking Panic,” American Economic Review 87, 863-83.
Calomiris, C. & J. Mason (2003), “Fundamentals, Panics and Bank Distress During the Great Depression: the June 1932 Chicago Banking Panic,” American Economic Review 93, 1615-47.
Camerer (2008), “Behavioral Game Theory”, The New Palgrave Dictionary of Economics, 2nd ed. S.N. Durlauf & L.E. Blume (Eds.), vol. 1, 444-448, Palgrave Macmillan.
Chakravarty, S. M. Fonseca & T. Kaplan (2013), “An Experiment on the Causes of Bank Run Contagions,” mimeo.
Chancellor, E. (1999), Devil Take the Hindmost: A History of Financial Speculation, New York: Farrar, Straus & Giraux.
Charness, G. & M. Dufwenberg (2006), “Promises & Partnership,” Econometrica 74, 1579-1601.
Crawford, V. M. Costa-Gomes & N. Iriberri (2013), “Structural Models of Nonequilibrium Strategic Thinking: Theory, Evidence & Applications,” Journal of Economic Literature 51, 5-62.
Dash, Mike (1999), Tulipomania: The Story of the World's Most Coveted Flower and the Extraordinary Passions it Aroused, Crown Publishers.
Dasgupta, A. (2004), “Financial Contagion through Capital Connections: A Model of the Origin and Spread of Bank Panics,” Journal of the European Economic Association 2, 1049-84.
De Long, B., A. Schleifer, L. Summers & R. Waldmann (1990, “Noise Trader Risk in Financial Markets,” Journal of Political Economy 98, 703-38.
Diamond, D. & P. Dybvig. (1983) “Bank Runs, Deposit Insurance and Liquidity.” Journal of Political Economy, 91, 401-419.
Dufwenberg, M., T Lindqvist & E Moore (2005), “Bubbles & Experience: An Experiment”, American Economic Review 95, 1731-36.
Dufwenberg, M. & G. Kirchsteiger (2004), “A Theory of Sequential Reciprocity,” Games & Economic Behavior 47, 268-98.
Fisher, E. & F. Kelly (2000), “Experimental Foreign Exchange Markets.” Pacific Economic Review 5 365-87.
Financial Crisis Inquiry Report (2011), National Commission on the Causes of the Financial & Economic Crisis in the United States (The Financial Crisis Inquiry Commission, FCIC; chair: Phil Angelides,10 members. [http://www.gpo.gov/fdsys/pkg/GPO-FCIC/pdf/GPO-FCIC.pdf]
Friedman, M. & A. Schwartz (1963), A Monetary History of the United States, 1867-1960, Princeton University Press.
Garber, P. (2000), Famous First Bubbles: The Fundamentals of Early Manias, MIT Press.
Garratt, R. & T. Keister (2009), “Bank Runs as Coordination Failures: An Experimental Study,” Journal of Economic Behavior & Organization 71, 300-17.
Gilovich, T., R. Vallone & A. Tversky (1985), “The Hot Hand in Basketball: On the Misperception of Random Sequences,” Cognitive Psychology 17, 295-314.
Goldstein, I. & A. Pauzner (2005), “Demand-Deposit Contracts and the Probability of Bank Runs,” Journal of Finance 60, 1293-27.
Gorton, G. (1988), “Banking Panics & Business Cycles,” Oxford Economic Papers 40, 751-81.
Green, E. & P. Lin (2003), “Implementing Efficient Allocations in a Model of Financial Intermediation,” Journal of Economic Theory 109, 1-23.
Haruvy, E., Y. Lahav & C. Noussair (2007), “Traders’ Expectations in Asset Markets: Experimental Evidence,” American Economic Review 97, 1901-20.
Haruvy, E., & Noussair, C. (2006), “The Effect of Short-Selling on Bubbles and Crashes in Experimental Spot Asset Markets,” Journal of Finance 61, 1119-57.
Heinemann, F., R. Nagel & P. Ockenfels (2004), “The Theory of Global Games on Test: Experimental Analysis of Coordination Games with Public and Private Information,” Econometrica 72, 1583-99.
Hussam, R. D. Porter & V. Smith (2008), “Thar She Blows: Can Bubbles Be Rekindled with Experienced Subjects?” American Economic Review 98, 924-37.
Kagel, J. & A. Roth (eds.) (1995), Handbook of Experimental Economics, Princeton Univ Press.
Kahneman, D. & A Tversky, eds. (2000), Choices, Values, and Frames, Cambride Univ Press.
Kindleberger, C. (2001) Manias, Panics & Crashes: A History of Financial Crisis, Wiley.
King, R., V. Smith, A. Williams & M. Van Boening (1993), “The Robustness of Bubbles and Crashes in Experimental Stock Markets,” in R. Day & P. Chen (eds.), Nonlinear Dynamics and Evolutionary Economics. Oxford University Press.
Kirchler, M., J. Huber & T. Stöckl (2012) “Thar She Bursts: Reducing Confusion Reduces Bubbles,” American Economic Review 102, 865-83.
Kiss, H.J., I. Rodriguez-Lara & A. Rosa-Garcia (2011), “On the Effects of Deposit Insurance and Observability on Bank Runs: An Experimental Study,” Discussion Paper in Economic Behavior 02/11, ERI-CES, University of Valencia. Forthcoming in Journal of Money, Credit, & Banking.
Kiss, H.J., I. Rodriguez-Lara & A. Rosa-Garcia (2012), “Do Social Networks Prevent Bank Runs?” Discussion Paper in Economic Behavior 08/12, ERI-CES, University of Valencia.
Klos, A. & N. Sträter (2012), “How Strongly Do Players React to Changes in Payoff Parameters in an Experimental Bank Run Game?” mimeo.
Krugman, P. (2010/Jan. 11), “Too Big to Fail,” The Conscience of a Liberal, New York Times.
Lei, V., C. Noussair & C. Plott (2001), “Nonspeculative Bubbles in Experimental Asset Markets: Lack of Common Knowledge of Rationality vs. Actual Irrationality,” Econometrica 69, 831+.
Lei, V. & F. Vesely (2007), “Market Efficiency: Evidence from a No-Bubble Asset Market Experiments,” Pacific Economic Review 14, 246-58.
Madiès, P. (2006), “An Experimental Exploration of Self-Fulfilling Banking Panics: Their Occurrence, Persistence, and Prevention,” Journal of Business 79, 1831-1866.
Minsky, H. (1975), John Maynard Keynes. Columbia University.
Minsky, H. (1992), “The Financial Instability Hypothesis,” The Jerome Levy Economics Institute of Bard College, Working Paper No. 74, May 1992.
Mishkin, F. (1992), “Anatomy of a Financial Crisis,” J of Evolutionary Economics 2, 115-30.
Noussair, C., S. Robin & B. Ruffieux (2001), “Price Bubbles in Laboratory Assets Markets with Constant Fundamental Values,” Experimental Economics 4, 87-105.
Oechssler, J., C. Schmidt, W. Schnedler (2011), “On the Ingredients for Bubble Formation: Informed Traders & Communication,” Journal of Economic Dynamics & Control 35, 1831-51.
Peck, J. & K Shell (2000), “Equilibrium Bank Runs,” Journal of Political Economy 111, 103-23.
Peterson, S. (1993), “Forecasting Dynamics and Convergence to Market Fundamentals: Evidence from Experimental Asset Markets,” Journal of Economic Behavior & Organization 22, 269-84.
Porter, D. & V. Smith (1995), “Futures Contracting and Dividend Uncertainty in Experimental Asset Markets,” Journal of Business 68, 509-41.
Rabin, M (1993), “Incorporating Fairness into Game Theory & Economics,” American Economic Review 83, 1281-1302.
Rabin, M. (2002), “Inference by Believers in the Law of Small Numbers,” Quarterly Journal of Economics 117, 775-816.
Rochet, J.-C. & X. Vives (2004), “Coordination Failures and the Lender of Last Resort: Was Bagehot Right After All?,” Journal of the European Economic Association 2, 1116-47.
Ross, S. (2008), “Finance”, The New Palgrave Dictionary of Economics, 2nd ed. S.N. Durlauf & L.E. Blume (Eds.), vol. 3, 314-333, Palgrave Macmillan.
Schotter, A. & T. Yorulmazer (2009), “On the Dynamics and Severity of Bank Runs: An Experimental Study,” Journal of Financial Intermediation 18, 217-241.
Shiller, R. (2000), Irrational Exuberance, Princeton University Press.
Smith, V. (1962), “An Experimental Study of Competitive Market Behavior,” Journal of Political Economy 70, 111-37.
Smith, V., G. Suchanek & A. Williams (1988), “Bubbles, Crashes and Endogenous Expectations in Experimental Spot Asset Markets,” Econometrica 56, 1119-51.
Stöckl, T., J. Huber & M. Kirchler (2010) “Bubble Measures in Experimental Asset Markets,” Experimental Economics 13, 284-98.
Søberg, M. (2005), The Duhem-Quine Thesis and Experimental Economics: A reinterpretation,” Journal of Economic Methodology 12, 581-97.
Summers, L. (2000), “International Financial Crisis: Causes, Prevention, and Cures,” American Economic Review 90, 1-15.
Summers, L. (2008), “Learning from and Responding to Financial Crisis,” Arthur M. Okun Lecture delivered at Yale University. [http://wn.com/diamond_dybvig_model]
Trautmann, S. & R. Vlahu (2012), “Strategic Loan Defaults and Coordination: An Experimental Analysis,” Journal of Banking & Finance 37, 747-60.
Van Boening, M., A. Williams & S. LaMaster (1993), “Price Bubbles and Crashes in Experimental Call Markets.” Economics Letters 41, 179-85.
van Damme, E. (1994), “Banking: A Survey of Recent Microeconomic Theory,” Oxford Review of Economic Policy 10, 14-33.
Wang, J. (2008), “Finance (New Developments)”, The New Palgrave Dictionary of Economics, 2nd ed. S.N. Durlauf & L.E. Blume (Eds.), vol. 3, 333-358, Palgrave Macmillan.
Wicker, E. (1996), The Banking Panics of the Great Depression, Cambridge University Press.
Wicker, E. (2000), Banking Panics of the Gilded Age, Cambridge University Press.
Fotnotar
University of Arizona, University of Gothenburg, CESifo Network; martind@eller.arizona.edu
For a different breakdown, consider the list of chapters of the1995 Handbook, indicating key streams focus up till then: Public Goods (author: J. Ledyard), Coordination Problems (J. Ochs), Bargaining Experiments (A. Roth), Industrial Organization (C. Holt), Experimental Asset Markets (S. Sunder), Auctions (J. Kagel), Individual Decision Making (C. Camerer). A second volume, again edited by Kagel & Roth, is in progress; its chapters indicate main streams of post-1995 focus (info from Al Roth’s webpage): Introduction: The Last Ten+ Years (A. Roth), Political Economy (T. Palfrey), Voluntary Giving to Public Goods (L. Vesterlund), Learning & the Economics of Small Decisions (I. Erev & E. Haruvy), Field Experiments: Psychology & Economics in the Field (author TBD), Neuroeconomics (C. Camerer, J. Cohen, E. Fehr, P. Glimcher, D. Laibson), Other-Regarding Preferences (D. Cooper & J. Kagel), Auctions (J. Kagel & D. Levin), Macroeconomics (J. Duffy), Market Design (A. Roth).
Charlie Holt’s chapter in the Handbook, cited in footnote 4, is a good guide and entry to much of the literature.
The phrasing “do not initially know the data-generating process” suggests B&T have in mind settings where the process is not only unknown to a decision maker but also independent of his decisions. Of course, in The Bubbles Example, the second assumption does not hold. Nevertheless, the underlying psychology may be the same. The idea is furthermore reminiscent of Hyman Minsky’s (1975, 1992) “Financial Instability Hypothesis”.
D&D work with an infinite number of customers, but the intuition is the same. Readers familiar with risk aversion concepts will notice that the utility function exhibits a constant relative risk aversion coefficient equal to 2; it has the properties of u(w) = w1-a/(1-a), with a = 2. The essence of the example would go through for any a > 1.
With n = 6 the bank gets deposits of 6×1 in period 0. It pays out 3×(1+r) in period 1 and is then left with 3×(1-r) which by period 2 grows to 3×(1-r)×4, which is shared equally by its three remaining customers.
7/8 is in fact the best outcome possible as r = 1/3 is the optimal level of r. To see this, take the derivatative with respect to r of the expected utility expression ½ × (2-2/(1+r)) + ½ × (2-2/((1-r)×4), set it equal to 0, and solve for r.
D&D assume that whenever multiple customers simultaneously attempt to withdraw funds, a “sequential service constraint” applies such that it is randomly determined who gets stipulated return (1+r = 4/3, in our example), up until when the bank runs out of funds. Note that 4×(4/3)+(2/3)+0 = 18/3 = 6 = the total period 0 deposits.
The conclusions of the the Financial Crisis Inquiry Report (2011, p. xvii) echoe this: “Despite the expressed view of many on Wall Street and in Washington that the crisis could not have been foreseen or avoided, there were warning signs. The tragedy was that they were ignored or discounted. There was an explosion in risky subprime lending and securitization, an unsustainable rise in housing prices, widespread reports of egregious and predatory lending practices, dramatic increases in household mortgage debt, and exponential growth in financial firms’ trading activities, unregulated derivatives, and short-term “repo” lending markets, among many other red flags. Yet there was pervasive permissiveness; little meaningful action was taken to quell the threats in a timely manner.”
With reference to my D&D account (III-B), assume that for each unit deposited with the bank it has to hold cash reserves k ≤ 1, and if an invested unit is pulled back in period , its value is y ≤ 1. With n depositors, the amount available for period 1 withdrawals is n×(k+(1-k)×y). Section III-B considered the case y = 1, where n×(k+(1-k)×y) = n, independent of k. If y < 1 then n×(k+(1-k)×y) < n, and the payoffs of various strategy profiles now depend on k.